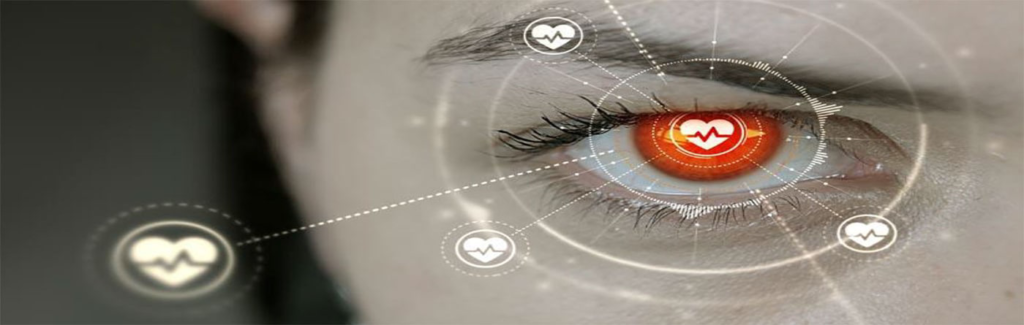
For prevention of vision loss and promotion of quality of life, it is crucial to detect and diagnose eye diseases at an early stage so that an appropriate management strategy can be made. Integration of Artificial Intelligence (AI) in Ophthalmology has a great potential to create a revolutionary change in the pattern of disease diagnosis and significant clinical impact.
Artificial intelligence (AI), the new era has evolved and is ruling technology in many aspects which in turn plays a vital role in the field of medicine including Ophthalmology. AI refers to a machine that imitates the human’s thought process and behavioural pattern. AI is a branch of computer science that involves creating machines to replicate human intelligence. The application of this forthcoming technology makes the field of ophthalmology digitalized, which prospects the accessibility, availability, and productivity of existing overall efficiency of eye care services, and it avidly focused on diseases such as Diabetic retinopathy (DR), Glaucoma, Age-related macular degeneration, retinal vein occlusion, Cataract and Retinopathy of prematurity.
Aim of AI
- Detection of DR and glaucoma with fundus images, OCT images, visual fields, and other clinical data.
- Detection of the condition and referral before it worsens.
- It has also been applied to study various risk factors of the disease condition and the quality of life.
Classification of AI algorithms

Figure 1: Introduction of AI algorithms [Photo courtesy: Lu, W., Tong, Y., Yu, Y., Xing, Y., Chen, C., & Shen, Y. (2018). Applications of artificial intelligence in ophthalmology: general overview. Journal of ophthalmology, 2018.]
Machine learning
Machine learning (ML) is defined as a set of methods that automatically detect patterns in data and then incorporate this information to predict future data under uncertain conditions. Machine learning is further divided into Supervised and Unsupervised, which implies the labelling of the data provided for classification into disease and non-disease categories.
Deep learning
Deep learning is a subset of machine learning that uses an artificial neural network (ANN) structure which was inspired by the biological neural network (Visual cortex).

Figure 2: Convolutional neural network framework. (a) One or more filter functions reduce portions of the image (green box) into mathematical representations, creating a feature map (grey circle). (b) Pooling functions combine similar statistics from extracted features. (c) Fully connected layers are used in the classification process, in which each node is connected with every other node in the preceding layer. Multiple repetitions in multiple layers yield a final output. [Photo courtesy: Zheng, C., Johnson, T. V., Garg, A., & Boland, M. V. (2019). Artificial intelligence in glaucoma. Current opinion in ophthalmology, 30(2), 97-103.]

Figure 3: Potential applications of a DL solution for DR screening. Illustration of the application of a DL solution for DR screening using imaging, comparing existing clinical practice with a fully automated AI model (replacement) and a semiautomated AI model (triage). AI indicates artificial intelligence, DL, deep learning: DR, diabetic retinopathy. [Photo courtesy: Gunasekaran, D. V., & Wong, T. Y. (2020). Artificial intelligence in ophthalmology in 2020: a technology on the Cusp for translation and implementation.]

Figure 4: Proposed system for the detection of glaucoma. [Photo courtesy: Nayak, J., Acharya, R., Bhat, P. S., Shetty, N., & Lim, T. C. (2009). Automated diagnosis of glaucoma using digital fundus images. Journal of medical systems, 33(5), 337-346.]
Needs to be addressed
- Scarcity of ophthalmologists or trained persons for grading the retinal images.
- It is difficult for people from rural areas to undergo eye examinations.
- Follow-up is required for years, which might hinder the patient’s interest in participation.
To overcome this, automated imaging systems and software which can efficiently analyse the captured retinal images and are easily available to reach the patient.
AI IN EYE CARE:
1. Vision and Refractive Error
New machine learning-based diagnostic applications for refraction, vision correction, and surgical and post-surgical management has expanded rapidly. Example: the wavefront aberrometer. Recent software of Iterative Dichotomize Three (ID3) analyses refractive variables: tear production, lenticular powers, corneal curvatures, and age to provide final prescriptions for eyeglasses.
2. Cornea And Ocular Surface
An AI algorithm has been developed to detect corneal abnormalities using the principle of pattern detection through corneal topography for early detection and acceleration of conditions as keratoconus, Terrine’s corneal abnormalities. Researchers are using machine learning techniques to analyse and diagnose early patterns for dry eye disorders, most of which will be driven by AI software and AI robotics, respectively.
3. Anterior Segment
Recent Deep learning technologies have been helpful to diagnose and make treatment decisions for congenital cataracts. Usage of AI robotic machines also has been evolving in cataract surgeries by planning surgery, calculating location of incision, minimizing error margin, and collating feedback for future operations. An AI Bayesian logic network is also being used for first or second diagnoses of various systemic conditions associated with uveitis.
4. Retina
AI has now become a valuable clinical tool in the case of Diabetic retinopathy for identifying and grading its severity. Machine learning is now also used for prediction of best correctable vision with anti-VEGF therapies in Age-related macular degeneration as well as in quantitative characterization of neovascular lesions in large-scale clinical spectraldomain optical coherence tomography datasets.
5. Glaucoma And Neuro Ophthalmic Disorders
AI-based software makes comparisons of thousands of healthy and glaucomatous optic nerve head images and hence allows machine learning to identify glaucomatous changes. Applying Machine Learning methods can improve the detection of pre-perimetric glaucoma Visual Fields from healthy Visual Fields significantly.
6. Immunotherapy, Genetics And Stem Cell Therapies
Recently, AI technologies and Robotics have been evolving in genomic medicine and health. AI can effectively play role in cancer diagnosis, therapies, immunotherapies, genetic therapies, and stem cell therapies for various congenital and acquired eye disorders causing blindness.
Example AI Model in Retinopathy:
What is Diabetic Retinopathy?
Diabetic Retinopathy is an eye disease associated with long-standing diabetes. This happens when high blood sugar levels cause damage to blood vessels in the retina. These blood vessels can swell and leak. Or they can close, stopping blood from passing through. Sometimes abnormal new blood vessels grow on the retina. All of these changes can steal your vision
How can we help?
Currently, detecting Diabetic Retinopathy (DR) is a time-consuming and manual process that requires a trained clinician to examine and evaluate digital colour fundus photographs of the retina. Expertise and equipment required to diagnose the disease often lacks in the areas where rate of diabetes in local populations is high and DR detection is most needed. As the number of individuals with diabetes continues to grow, the infrastructure needed to prevent blindness due to DR will become even more insufficient.
We need a comprehensive and automated method for DR screening. With colour fundus photography as input, we need to detect whether eye has DR or not automatically. If there is DR, we can go even further and rate how severe the disease is in the eye.




Requirements of AI for real-world transformation
- Good and ample number of clinical datasets.
- Eminent business and product management team, funding, and research team to conduct further research.
Conclusion
AI is expected to make a great impact in healthcare by using the clinical datasets to narrow down the diagnosis and to set a clinical pattern to assist and aid the clinician inevitably. Future research is required to know the viability of algorithms applied in the clinical setting to rule out the development and its outcomes in comparison with ophthalmologic practice.
#AI #ML #DL #Image Processing #Retinopathy #Eyecare #AIforEye
#AI #ML #DL #WEB 3.0 #third gen internet #datascience